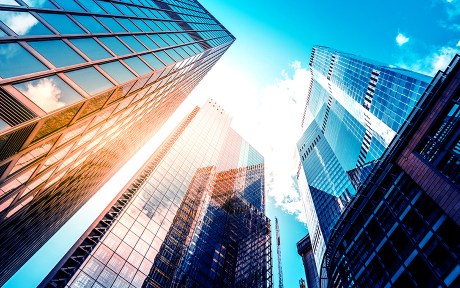
The first post in this series discussed the potential exposure of banks to the open-end funds sector, by virtue of commonalities in asset holdings that expose banks to balance sheet losses in the event of an asset fire sale by these funds. In this post, we summarize the findings reported in a recent paper of ours, in which we expand the analysis to consider a broad cross section of non-bank financial institution (NBFI) segments. We unveil an innovative monitoring insight: the network of interconnections across NBFI segments and banks matters. For example, certain nonbank institutions may not have a meaningful asset overlap with banks, but their fire sales could nevertheless represent a vulnerability for banks because their assets overlap closely with other NBFIs that banks are substantially exposed to.
Network Externalities in Fire-Sale Shocks
We expand the analysis in part one of this series to consider simultaneously twelve distinct nonbank institution types. Expanding the cross section of NBFI types permits us to consider the complexity of interconnections in the financial ecosystem, where banks and nonbanks operate in multiple markets. In turn, this consideration enables us to unveil the existence of important network externalities in the transmission of fire-sale shocks.
As an example of network effects, suppose we are interested in monitoring bank vulnerabilities with respect to a given NBFI sector S. In addition to tracking the similarity in asset holdings between banks and entities in S, it may also be important to know how central such entities might be—in terms of asset holding interconnections—within the diverse network of all the NBFI types. This is because entities in S may carry a well-diversified portfolio of assets, implying a significant asset overlap with many other NBFI market segments. This broad asset overlap implies a higher likelihood of experiencing distress if any of the other NBFI segments initiate fire sales, which in turn means a higher likelihood that entities in S transmit shocks to banks. Moreover, if central in the NBFI network, fire sales from entities in S could themselves impose distress on a broad set of other NBFIs. In sum, sector S could be a potentially important source of bank vulnerabilities due to its centrality in the NBFI network, even if on a stand-alone basis, their fire sale impact on banks were limited.
Analysis of NBFI Networks
We collect information on the asset composition of NBFI segments using the quarterly Financial Accounts of the United States (Z.1) issued by the Federal Reserve Board, commonly known as the Flow of Funds. As Flow of Funds data is reported only as an aggregate for a given sector type, we trade off data granularity with breadth of coverage when examining the network. With aggregate data, while we lose finer detail, we gain the ability to uncover (complex) mechanisms of transmission and amplifications and generate innovative monitoring insights. The table below shows the cross-holding matrix by institution type and asset from the 2021: Q4 Flow of Funds.
Cross-Holding Matrix From the 2021:Q4 Flow of Funds
Amounts in Billions of U.S. Dollars | Equity | Agency MBS | Bank Loan | Open Market Paper | Corp Bond | Gov’t Bond | Muni Bond | Cash | Total |
Banks | 54 | 3,883 | 12,631 | 0 | 888 | 1,641 | 643 | 4,221 | 23,962 |
P&C insurers | 643 | 136 | 28 | 4 | 702 | 188 | 289 | 142 | 2,133 |
Life insurers | 133 | 231 | 808 | 23 | 3,266 | 175 | 222 | 141 | 4,998 |
Money market funds | 0 | 410 | 0 | 226 | 7 | 1,815 | 111 | 2,640 | 5,208 |
Mutual funds (equity) | 14,270 | 0 | 0 | 26 | 0 | 0 | 0 | 190 | 14,486 |
Mutual funds (bonds) | 0 | 492 | 131 | 10 | 2,485 | 1,447 | 900 | 73 | 5,537 |
Mutual funds (hybrid) | 1,264 | 49 | 13 | 3 | 250 | 145 | 90 | 24 | 1,840 |
Exchange-traded funds | 5,804 | 0 | 0 | 0 | 800 | 331 | 83 | 39 | 7,057 |
Mortgage REITs | 0 | 168 | 0 | 0 | 12 | 0 | 0 | 17 | 197 |
Broker-dealers | 234 | 54 | 0 | 16 | 15 | 99 | 13 | 1,396 | 1,827 |
Finance companies | 0 | 0 | 1,026 | 0 | 99 | 0 | 0 | 57 | 1,182 |
Hedge funds | 1,140 | 8 | 181 | 0 | 474 | 165 | 15 | 227 | 2,210 |
Pension funds | 4,932 | 321 | 23 | 44 | 1,312 | 695 | 0 | 666 | 7,993 |
Total | 28,475 | 5,753 | 14,840 | 354 | 10,308 | 6,701 | 2,367 | 9,832 |
Note: Data adjusted to break Mutual Funds down into three subtypes.
The data shows considerable variation in terms of relative size and portfolio of asset holdings in the cross section of institution types, suggesting heterogeneity in terms of both first-round fire-sale effects, but also hard-to-guess, second-round losses following on from the first-round losses.
We apply the same methodology used in the companion post, and in previous Liberty Street Economics posts here and here, based on work by Greenwood, Landier, and Thesmar (2015). The table below shows the impact on banks from hypothetical first-round and second-round fire sales following from assumed losses for each institution type. The third through fifth columns display the first-round effects expressed as, respectively, the dollar loss on the aggregate balance sheet of banks, the loss as a percentage of banks’ aggregate equity capital, and the rank order of each NBFI institution type in terms of banks’ losses. Finance companies and life insurance companies create the most first-round bank losses, followed by mutual funds (bonds), hedge funds, and pension funds. As in Greenwood, Landier, and Thesmar (2015), an institution’s importance to banks (their “systemicness”) depends on multiple factors: size (how many dollars of assets it sells), interconnectedness (whether it holds asset classes that banks also hold), and the liquidity of holdings (for a given sale amount, more illiquid assets will have a greater price impact, resulting in greater losses for holders of those assets).
First and Second Round Losses for Banks
First-Round Loss | Second-Round Loss | |||||||||||||
Institution Type | Size (Billions of U.S. Dollars) | Billions of U.S. Dollars | Bank Capital (Percent) | Rank | Billions of U.S. Dollars | Bank Capital (Percent) | Rank | Second-Round Share (Percent) | ||||||
Banks | 23,962 | |||||||||||||
P&C insurers | 2,133 | -2.2 | -0.12 | 7 | -18.6 | -0.97 | 7 | 89 | ||||||
Life insurers | 4,998 | -21.3 | -1.11 | 2 | -45.9 | -2.39 | 4 | 68 | ||||||
Money market funds | 5,208 | -2.6 | -0.14 | 6 | -2.9 | -0.15 | 10 | 53 | ||||||
Mutual funds (equity) | 14,486 | -1.2 | -0.06 | 9 | -60.3 | -3.15 | 2 | 98 | ||||||
Mutual funds (bonds) | 5,537 | -8.9 | -0.46 | 3 | -68.6 | -3.58 | 1 | 89 | ||||||
Mutual funds (hybrid) | 1,840 | -1 | -0.05 | 10 | -12.3 | -0.64 | 8 | 93 | ||||||
Exchange-traded funds | 7,057 | -1.7 | -0.09 | 8 | -43.4 | -2.27 | 5 | 96 | ||||||
Mortgage REITs | 197 | -0.3 | -0.02 | 11 | -0.4 | -0.02 | 12 | 57 | ||||||
Broker-dealers | 1,827 | -0.2 | -0.01 | 12 | -1.5 | -0.08 | 11 | 86 | ||||||
Finance companies | 1,182 | -22.3 | -1.16 | 1 | -10.3 | -0.54 | 9 | 32 | ||||||
Hedge funds | 2,210 | -4.6 | -0.24 | 4 | -23.6 | -1.23 | 6 | 84 | ||||||
Pension funds | 7,993 | -3.3 | -0.17 | 5 | -52.8 | -2.75 | 3 | 94 |
For the first round of fire-sale losses, what matters is whether an institution holds asset classes that banks also hold. If we include other institutions’ reactions, it also matters whether an institution holds asset classes held by institutions that hold asset classes that banks also hold. To examine these derived effects, we simulate a second round of fire sales within our framework, where we now consider the losses incurred by every institution type to each of the first-round fire sales, and the resulting second-round fire sales. The second half of the above table shows the impact on banks from the aggregation of second-round fire sales. Mutual Funds (Bonds) are the highest ranked as vectors of shock amplification. Within our framework, corporate bonds are the most broadly held asset class and thus a firesale concentrated in bonds has a large second-round effect. The second to fourth rank are now taken by mutual funds (equity), pension funds, and once again life insurance companies. In addition to their size and the nature of their holdings, life insurers’ diversification results in high connectedness. Similarly, because of a lack of connectedness, finance companies’ rank drops from first to ninth. While their loan sales can hurt banks directly, due to their portfolio concentration they are less likely to hurt others, and thus the additional induced fire sales are relatively less severe.
Finally, the last column of the table above shows the “network multiplier,” defined as the ratio of the second-round loss over the total (first- plus second-round) loss. The ratio by construction ranges between 0 and 100 percent. The fairly large estimates in the cross section thus suggest that if we only focus on the direct fire-sale effect of a given NBFI segment onto banks, we are missing an important and potentially dominant component of the total effect.
Final Words
We have documented the potential vulnerabilities of banking institutions to fire sales initiated in the NBFI sector when considering both direct spillovers (fire sales of assets that are also held by banks) and indirect, “second-round” spillovers (fire sales that induce further fire sales by other NBFIs that in turn hurt banks). Our analysis sheds light on the intricate network of spillover exposures in the U.S. financial system and identifies a rank ordering of monitoring priorities across NBFI segments. Our framework thus supports the creation of novel monitoring tools.

Nicola Cetorelli is the head of Non-Bank Financial Institution Studies in the Federal Reserve Bank of New York’s Research and Statistics Group.
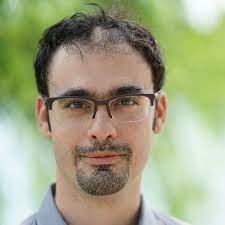
Mattia Landoni is a senior financial economist at the Federal Reserve Bank of Boston.
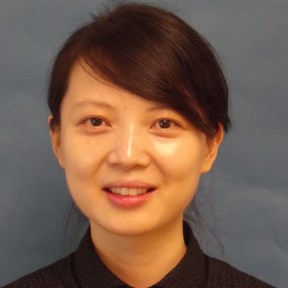
Lina Lu is a senior financial economist at the Federal Reserve Bank of Boston.
How to cite this post:
Nicola Cetorelli, Mattia Landoni, and Lina Lu, “Monitoring Banks’ Exposure to Nonbanks: The Network of Interconnections Matters,” Federal Reserve Bank of New York Liberty Street Economics, April 18, 2023, https://libertystreeteconomics.newyorkfed.org/2023/04/monitoring-banks-exposure-to-nonbanks-the-network-of-interconnections-matters/.
Disclaimer
The views expressed in this post are those of the author(s) and do not necessarily reflect the position of the Federal Reserve Bank of New York or the Federal Reserve System. Any errors or omissions are the responsibility of the author(s).
We would like to start by thanking the reader for his comment. As our work is a simulation of, in a sense, the whole financial system, we welcome open discussion of our assumptions by experts from every segment of the financial system – not only mutual funds.
Specifically, the reader is questioning the plausibility of our assumption that bond funds under stress would liquidate their portfolio proportionally to their holdings (“vertical slicing”), pointing out that this assumption results in sales that are four times as large as the actual experience of U.S. core bond funds in March 2020. We are not immediately able to review the figures shown by the reader, but we believe that our analysis is valuable regardless of this observation for two reasons.
First, precisely because the magnitudes in our simulations depend on assumptions, we have made these assumptions transparent and, in our paper, we clearly emphasize the value of focusing on ranks (relative comparisons across segments) rather than absolute magnitudes.
Second, “vertical slicing” seems to be what funds want to do, what regulators recommend, and what usually happens. The reader should not take our word for it. For example, BlackRock (2021) states: “Funds aim to meet investor redemptions by selling a part of each market segment the fund is invested in (e.g., by maturity, rating, and industry sector) in order to maintain risk constant positions over time across the portfolio, and not by accessing cash or liquid asset buffers. Regulation and best practice liquidity management standards reflect this, and recent evidence shows funds successfully met redemptions in March by using this approach.” In addition to the research noted by BlackRock, a recent academic study published in the Journal of Financial and Quantitative Analysis (Jiang, Li, and Wang, 2021) finds that U.S. open-end funds tend to use vertical slicing in times of stress. This finding has been reproduced for Canadian funds (Bank of Canada, 2018) and for European funds specifically during the COVID crisis (ESMA, 2021). Finally, a report by IOSCO (2018) also recommends vertical slicing as a best practice, especially in times of stress, because “if highly liquid assets are sold first, rather than applying a pro-rata approach, to pay redemptions orders, the remaining investors would be harmed, if the fund is left with disproportionate exposure to illiquid assets.” Taken together, these observations suggest that, for the purposes of our study, it is more sensible – and more credible – to use assumptions reflecting standard norms.
References Bank of Canada, 2018. How do Canadian Corporate Bond Mutual Funds Meet Investor Redemptions? [https://www.banqueducanada.ca/2018/05/note-analytique-personnel-2018-14/]
BlackRock, 2021. Liquidity Risk Management is Central to Open Ended Funds, Addendum. [https://www.blackrock.com/corporate/literature/whitepaper/viewpoint-addendum-lessons-from-covid-liquidity-risk-management-is-central-to-open-ended-funds-january-2021.pdf]
ESMA, 2021. Fund stress simulation in the context of COVID-19. [https://www.esma.europa.eu/sites/default/files/trv_2021_1-fund_stress_simulation_in_the_context_of_covid19.pdf]
IOSCO, 2018. OEF Liquidity and Risk Management – Good Practices and Issues for Consideration. [https://www.iosco.org/library/pubdocs/pdf/IOSCOPD591.pdf]
The blog’s conclusions depend on three underlying fundamental mechanisms: A segment’s asset holdings and how an initial shock translates into asset sales by that segment; how asset prices respond to these sales; and how the valuation shocks to these assets lead to further asset sales across all segments. Each of these poses a unique challenge for any modeler, especially when contemplating the complexity and drivers of these mechanisms and how they change over time.
Of course, modelling efforts need to abstract and focus on the pertinent features of the economy, but as in their 2016 and 2021 analyses, the authors unfortunately favored excessive abstraction over capturing reality to the detriment of their conclusions.
To offer a concrete example, the analysis assumes that mutual funds in response to an initial shock sell a proportional slice of their portfolio. But this is not consistent with reality. For example, in March 2020, core bond mutual funds had outflows of $125 billion. But in meeting these redemptions, they sold far less than a proportionate slice of their investment grade corporate bonds: 6.5% of the $125 billion in outflows versus 28.1% of their $2,779 billion holdings (https://www.ici.org/viewpoints/22-view-bondfund-survey-4). Hence, no matter the assumption about how the sales of these bonds affect prices, the imputed impact of the initial shock is already overstated by more than 300%!
As a result, it’s difficult to give credence to any of the blog’s conclusions.