Can option traders take a square root? Surprisingly, maybe not. This post shows that VIX futures prices exhibit significant deviations from their option-implied upper bounds—the square root of variance swap forward rates—thus violating the law of one price, a fundamental concept in economics and finance. The deviations widen during periods of market stress and predict the returns of VIX futures. Just as the stock market struggles with multiplication, the equity volatility market appears unable to take a square root at times.
The Law of One Price in Equity Volatility Markets
Job Seekers’ Beliefs and the Causes of Long‑Term Unemployment
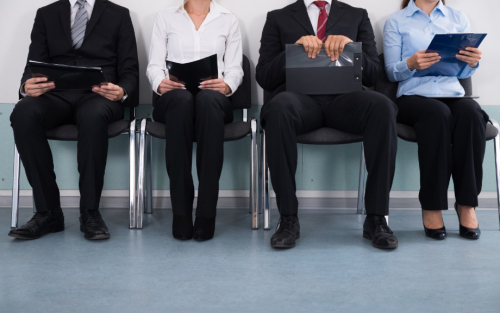
In addition to its terrible human toll, the COVID-19 pandemic has also caused massive disruption in labor markets. In the United States alone, more than 25 million people lost their jobs during the first wave of the pandemic. While many have returned to work since then, a large number have remained unemployed for a prolonged period of time. The number of long-term unemployed (defined as those jobless for twenty-seven weeks or longer) has surged from 1.1 million to almost 4 million. An important concern is that the long-term unemployed face worse employment prospects, but prior work has provided no consensus on what drives this decline in employment prospects. This post discusses new findings using data on elicited beliefs of unemployed job seekers to uncover the forces driving long-term unemployment.
Discretionary and Nondiscretionary Services Expenditures during the COVID‑19 Recession
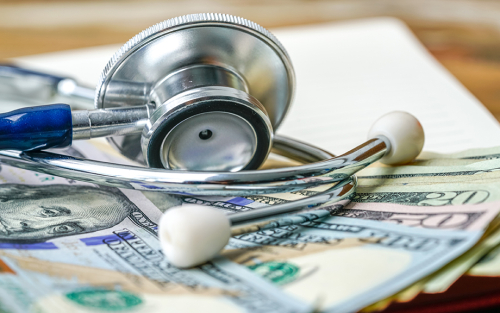
The coronavirus pandemic and the various measures to address it have led to unprecedented convulsions to the U.S. and global economies. In this post, I examine those extraordinary impacts through the lens of personal consumption expenditures on discretionary and nondiscretionary services, a framework I developed in a 2011 post (and subsequently employed in 2012, 2014, and 2017). In particular, I show that there were exceptional declines in both services categories during the spring; their recoveries, however, have displayed notably different patterns in recent months, with nondiscretionary services expenditures nearly back to their prior level and discretionary services expenditures seemingly stalled well below their pre-pandemic peak.
Understanding the Racial and Income Gap in COVID‑19: Public Transportation and Home Crowding
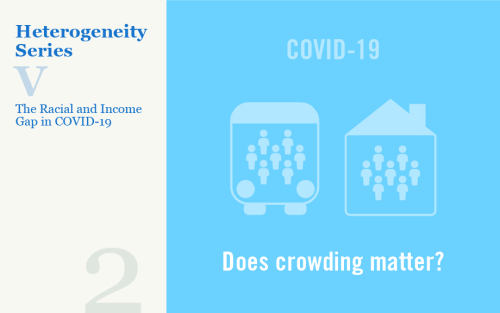
This is the second post in a series that aims to understand the gap in COVID-19 intensity by race and income. In our post yesterday, we looked at how comorbidities, uninsurance rates, and health resources may help to explain the race and income gap observed in COVID-19 intensity. We found that a quarter of the income gap and more than a third of the racial gap in case rates are explained by health status and system factors. In this post, we look at two factors related to indoor density—namely the use of public transportation and increased home crowding. Here, we will aim to understand whether these two factors affect overall COVID-19 intensity, whether the income and racial gaps of COVID can be further explained when we additionally include these factors, and whether and to what extent these factors independently account for income and racial gaps in COVID-19 intensity (without controlling for the factors considered in the other posts in this series).
Understanding the Racial and Income Gap in Covid‑19: Health Insurance, Comorbidities, and Medical Facilities
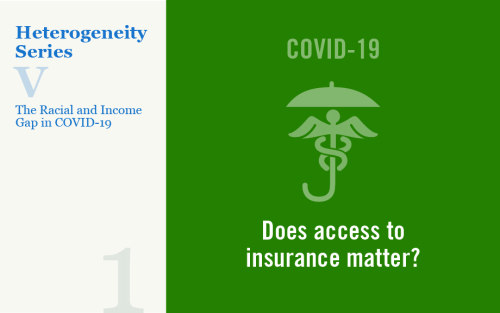
Our previous work documents that low-income and majority-minority areas were considerably more affected by COVID-19, as captured by markedly higher case and death rates. In a four-part series starting with this post, we seek to understand the reasons behind these income and racial disparities. Do disparities in health status translate into disparities in COVID-19 intensity? Does the health system play a role through health insurance and hospital capacity? Can disparities in COVID-19 intensity be explained by high-density, crowded environments? Does social distancing, pollution, or the age composition of the county matter? Does the prevalence of essential service jobs make a difference? This post will focus on the first two questions. The next three posts in this series will focus on the remaining questions. The posts will follow a similar structure. In each post, we will aim to understand whether the factors considered in that post affect overall COVID-19 intensity, whether the racial and income gaps can be further explained when we additionally include the factors in consideration in that post, and whether and to what extent the factors under consideration in that post independently affect racial and income gaps in COVID-19 intensity (without controlling for the factors considered in the other posts in this series).
The International Spillover of U.S. Monetary Policy via Global Production Linkages
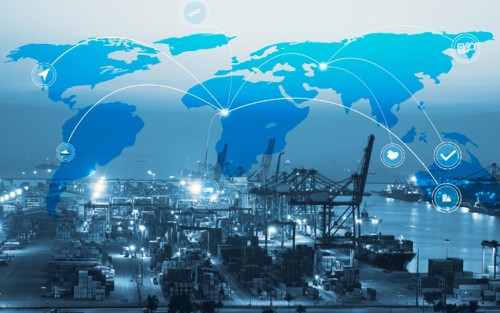
Julian di Giovanni describes work with Galina Hale that employs an empirical framework to quantify the role of the global production network in transmitting U.S. monetary policy across international stock markets.
Understanding the Impact of COVID‑19: The Top Five LSE Posts of 2020
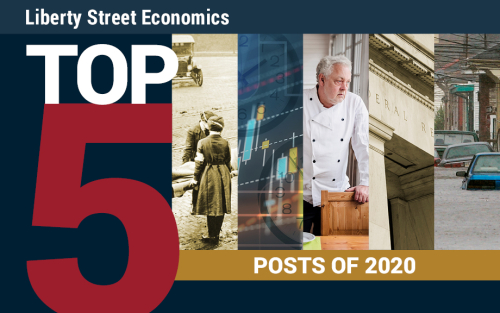
An annual tradition at Liberty Street Economics is to present our most-read posts of the year. Given the events of 2020, New York Fed economists and guest coauthors focused their analysis on the effects of the coronavirus pandemic, writing some seventy articles since March on the subject. Our leading posts, in terms of traffic, all touch on the theme in some way. Consider this space a hub for COVID-19 coverage for some time to come, and take a look back at the top five posts grabbing attention in 2020.
The New York Fed DSGE Model Forecast—December 2020
This post presents an update of the economic forecasts generated by the Federal Reserve Bank of New York’s dynamic stochastic general equilibrium (DSGE) model. We describe very briefly our forecast and its change since September 2020.
As usual, we wish to remind our readers that the DSGE model forecast is not an official New York Fed forecast, but only an input to the Research staff’s overall forecasting process. For more information about the model and variables discussed here, see our DSGE model Q & A. Note that interactive charts are now available for DSGE model forecasts.