COVID‑19 and Small Businesses: Uneven Patterns by Race and Income
Ruchi Avtar, Rajashri Chakrabarti, Davide Melcangi, Maxim Pinkovskiy, and Giorgio Topa
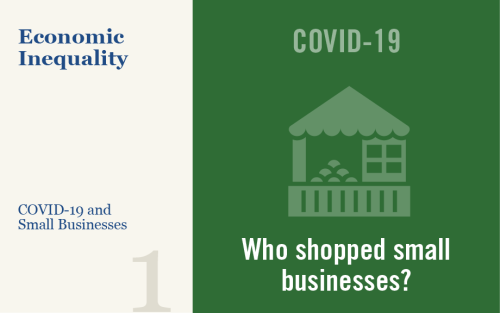
The COVID-19 pandemic resulted in one of the sharpest recessions and recoveries in U.S. history. As the virus spread over the country in a matter of weeks in March 2020, most states rapidly locked down nonessential economic activity, which plummeted as a result. As the first wave of COVID-19 subsided and people gradually learned to “live with the virus,” states reversed most of the initial lockdowns and economic activity rebounded. In our ongoing Economic Inequality series, we have explored many aspects of how the economic turmoil associated with COVID-19 differentially affected households. Here, we turn to small business experience. The first post in this three-part installment seeks to understand if there was variance in small business activity across counties that differed by income and race. In two companion posts, we investigate the experience of small businesses in terms of credit access, looking at characteristics of who received and who benefited from the Paycheck Protection Program (PPP). The analysis finds inequalities in PPP credit access as well as differences in the loan and recipient county characteristics for those who received loans through financial technology (fintech) providers versus other lenders.
Racial and Income Gaps in Consumer Spending following COVID‑19
Ruchi Avtar, Rajashri Chakrabarti, Maxim Pinkovskiy, and Giorgio Topa
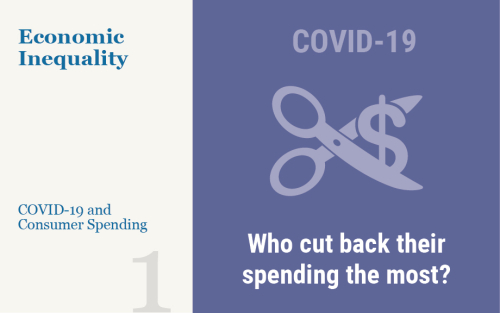
This post is the first in a two-part series that seeks to understand whether consumer spending patterns during the COVID-19 pandemic evolved differentially across counties by race and income. As the pandemic hit and social distancing restrictions were put into place in March 2020, consumer spending plummeted. Subsequently, as social distancing restrictions began to be relaxed later in spring 2020, consumer spending started to rebound. We find that higher-income counties had a considerably steeper decline and a shallower recovery than low-income counties did. The differences by race were also sizeable as the pandemic struck but became considerably more muted after summer of 2020. The decline and the recovery until the end of summer were sharper for majority-minority (MM) than majority nonminority (MNM) counties, while both sets of counties showed similar growth in spending after that. The second post in this series highlights the goods and services that were most adversely affected (or “constrained”) by the pandemic. Then, differentiating households by income, that post explores which households were more exposed to these pandemic-constrained expenditure categories.
Understanding the Racial and Income Gap in COVID‑19: Essential Workers
Ruchi Avtar, Rajashri Chakrabarti, and Maxim Pinkovskiy
This is the fourth and final post in this series aimed at understanding the gap in COVID-19 intensity by race and by income. The previous three posts focused on the role of mediating variables—such as uninsurance rates, comorbidities, and health resource in the first post; public transportation, and home crowding in the second; and social distancing, pollution, and age composition in the third—in explaining the racial and income gap in the incidence of COVID-19. In this post, we now investigate the role of employment in essential services in explaining this gap.
Investigating the Effect of Health Insurance in the COVID‑19 Pandemic
Rajashri Chakrabarti, Maxim Pinkovskiy, Will Nober, and Lindsay Meyerson
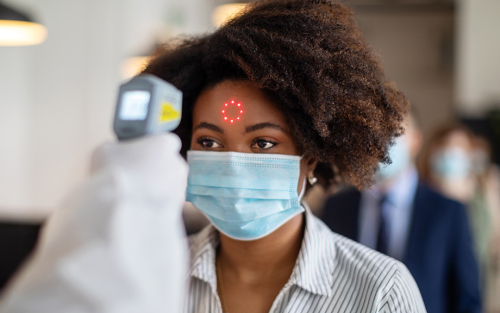
Does health insurance improve health? This question, while apparently a tautology, has been the subject of considerable economic debate. In light of the COVID-19 pandemic, it has acquired a greater urgency as the lack of universal health insurance has been cited as a cause of the profound racial gap in coronavirus cases, and as a cause of U.S. difficulties in managing the pandemic more generally. However, estimating the effect of health insurance is difficult because it is (generally) not assigned at random. In this post, we approach this question in a novel way by exploiting a natural experiment—the adoption of the Affordable Care Act (ACA) Medicaid expansion by some states but not others—to tease out the causal effect of a type of health insurance on COVID-19 intensity.
How Did State Reopenings Affect Small Businesses?
Rajashri Chakrabarti, Sebastian Heise, Davide Melcangi, Maxim Pinkovskiy, and Giorgio Topa
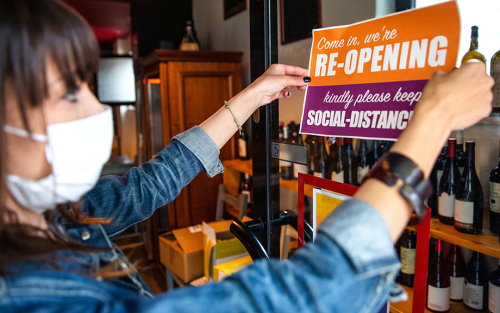
In our previous post, we looked at the effects that the reopening of state economies across the United States has had on consumer spending. We found a significant effect of reopening, especially regarding spending in restaurants and bars as well as in the healthcare sector. In this companion post, we focus specifically on small businesses, using two different sources of high-frequency data, and we employ a methodology similar to that of our previous post to study the effects of reopening on small business activity along various dimensions. Our results indicate that, much like for consumer spending, reopenings had positive and significant effects in the short term on small business revenues, the number of active merchants, and the number of employees working in small businesses. It is important to stress that we are not expressing any views in this post on the normative question of whether, when, or how states should loosen or tighten restrictions aimed at controlling the COVID-19 pandemic.
Did State Reopenings Increase Consumer Spending?
Rajashri Chakrabarti, Sebastian Heise, Davide Melcangi, Maxim Pinkovskiy, and Giorgio Topa
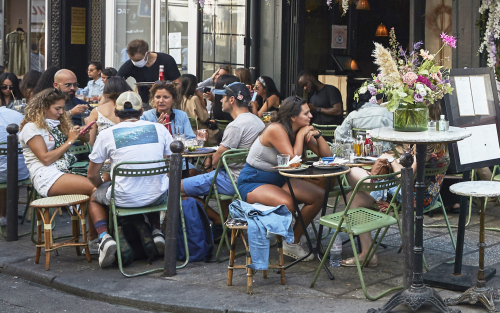
The spread of COVID-19 in the United States has had a profound impact on economic activity. Beginning in March, most states imposed severe restrictions on households and businesses to slow the spread of the virus. This was followed by a gradual loosening of restrictions (“reopening”) starting in April. As the virus has re-emerged over the last few weeks, a number of states have taken steps to reverse the reopening of their economies. For example, Texas and Florida closed bars again in June, and Arizona additionally paused operations of gyms and movie theatres. Taken together, these measures raise the question of how closures and reopenings affect consumer spending. In this post, we investigate how much consumer spending increased after the reopenings. It is important to stress that we are not expressing any views on the normative question of whether, when, or how states should loosen or tighten restrictions aimed at controlling the COVID-19 pandemic.
Debt Relief and the CARES Act: Which Borrowers Face the Most Financial Strain?
Rajashri Chakrabarti, Andrew Haughwout, Donghoon Lee, William Nober, Joelle Scally, and Wilbert van der Klaauw
In part I of our analysis, we studied the expected debt relief from the CARES Act on mortgagors and student debt borrowers. We now turn our attention to the 63 percent of American borrowers who do not have a mortgage or student loan. These borrowers will not directly benefit from the loan forbearance provisions of the CARES Act, although they may be able to receive some types of leniency that many lenders have voluntarily provided. We ask who these borrowers are, by age, geography, race and income, and how does their financial health compare with other borrowers.
Debt Relief and the CARES Act: Which Borrowers Benefit the Most?
Rajashri Chakrabarti, Andrew Haughwout, Donghoon Lee, William Nober, Joelle Scally, and Wilbert van der Klaauw
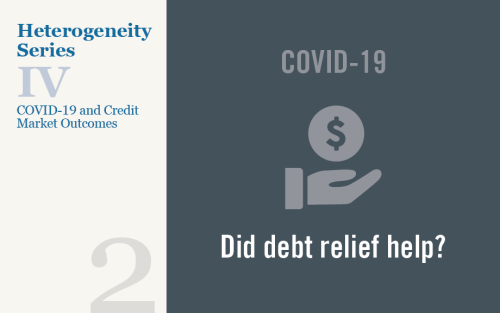
COVID-19 and associated social distancing measures have had major labor market ramifications, with massive job losses and furloughs. Millions of people have filed jobless claims since mid-March—6.9 million in the week of March 28 alone. These developments will surely lead to financial hardship for millions of Americans, especially those who hold outstanding debts while facing diminishing or disappearing wages. The CARES Act, passed by Congress on April 2, 2020, provided $2.2 trillion in disaster relief to combat the economic impacts of COVID-19. Among other measures, it included mortgage and student debt relief measures to alleviate the cash flow problems of borrowers. In this post, we examine who could benefit most (and by how much) from various debt relief provisions under the CARES Act.
Are Financially Distressed Areas More Affected by COVID‑19?
Rajashri Chakrabarti, William Nober, and Maxim Pinkovskiy
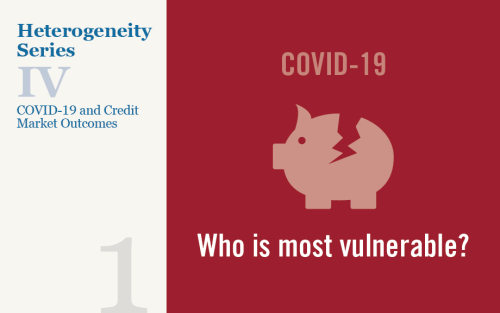
Building upon our earlier Liberty Street Economics post, we continue to analyze the heterogeneity of COVID-19 incidence. We previously found that majority-minority areas, low-income areas, and areas with higher population density were more affected by COVID-19. The objective of this post is to understand any differences in COVID-19 incidence by areas of financial vulnerability. Are areas that are more financially distressed affected by COVID-19 to a greater extent than other areas? If so, this would not only further adversely affect the financial well-being of the individuals in these areas, but also the local economy. This post is the first in a three part-heterogeneity series looking at heterogeneity in the credit market as it pertains to COVID-19 incidence and CARES Act debt relief.
Do College Tuition Subsidies Boost Spending and Reduce Debt? Impacts by Income and Race
Rajashri Chakrabarti, William Nober, and Wilbert van der Klaauw
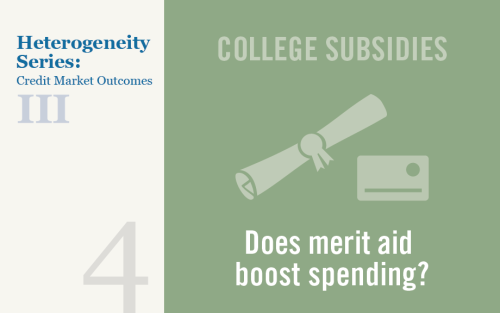
In an October post, we showed the effect of college tuition subsidies in the form of merit-based financial aid on educational and student debt outcomes, documenting a large decline in student debt for those eligible for merit aid. Additionally, we reported striking differences in these outcomes by demographics, as proxied by neighborhood race and income. In this follow-up post, we examine whether and how this effect passes through to other debt and consumption outcomes, namely those related to autos, homes, and credit cards. We find that access to merit aid leads to an immediate but temporary increase in eligible individuals’ consumption in these categories. The increase is followed by a decline in consumption and a reduction in total debt of these types in the longer term. Importantly, there are marked differences in these consumption and debt patterns across groups, as evident when we introduce proxies for demographic group using the income and racial composition of the students’ home neighborhoods of origin.